Reimplementing DiffEdit: A Journey Through Image Editing with Deep Learning
In this blog post, I'll share my experience reimplementing the DiffEdit paper, inspired by the fast.ai course "Practical Deep Learning for Coders." This project demonstrates how I used AI techniques to edit images, transforming a horse into a zebra using deep learning models.
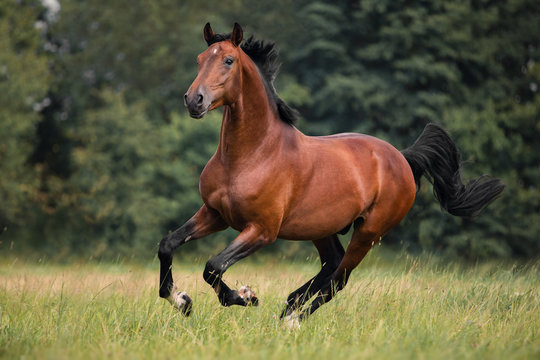
Step 1: Preparing the Image
I began by resizing my input image to 512x512 pixels, which is a standard size for many deep learning models.
Step 2: Semantic Segmentation with CLIP
Next, I used the CLIP model to create a semantic segmentation of my image, providing prompts like "horse" and "zebra" to identify the areas I wanted to edit.
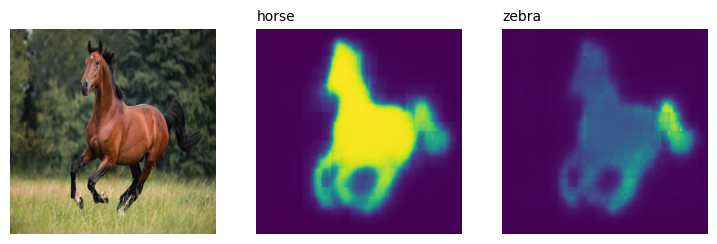
Step 3: Creating the Mask
I generated a mask by subtracting the "zebra" segmentation from the "horse" segmentation.
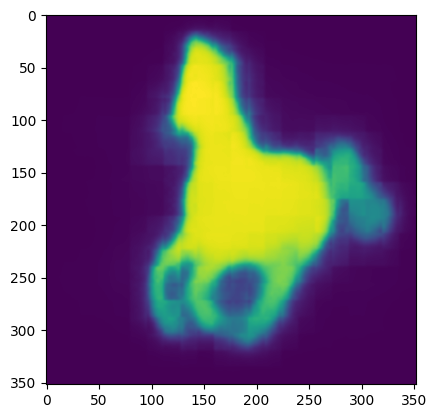
Step 4: Refining the Mask
To improve the quality of my edit, I applied Gaussian filtering to smooth the mask and then thresholded it.
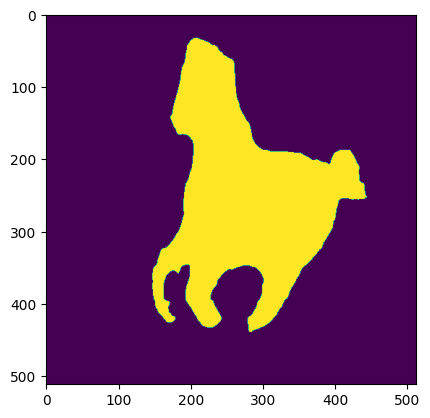
Step 5: Image Inpainting
Using the Stable Diffusion Inpainting model, I applied my edit with prompts describing what I wanted ("A zebra") and what I didn't want ("horse").

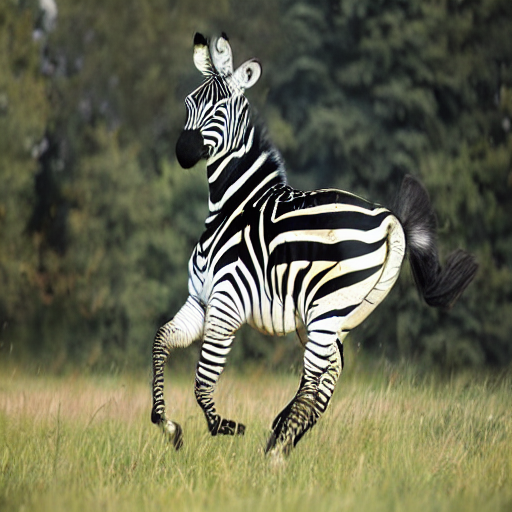
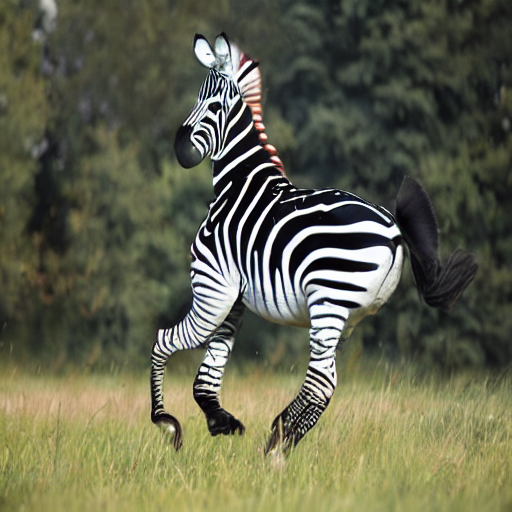
Results and Discussion
The final results showcase how I successfully transformed the original horse image into multiple variations of a zebra. This demonstrates the power of combining semantic segmentation with generative AI for targeted image editing.
Conclusion
My reimplementation of DiffEdit showcases the potential of deep learning in creative tasks. By leveraging models like CLIP and Stable Diffusion, I was able to perform complex image edits with relatively simple prompts.
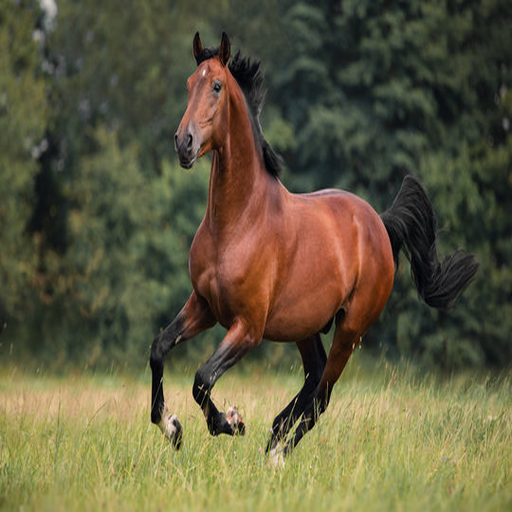
